Understanding Predictive Analytics
Predictive analytics refers to the use of statistical techniques, algorithms, and machine learning tools to analyze historical data and make informed predictions about future outcomes. Its primary purpose is to identify trends, anticipate events, and enhance decision-making processes across different sectors.
In today’s data-driven landscape, organizations harness predictive analytics to gain a competitive edge, improve operational efficiency, and enhance customer experiences. This forward-looking approach allows businesses to leverage their data assets, transforming raw information into actionable insights.
Its evolution can be traced back to its roots in statistics and data mining. Early forms of statistical analysis focused on descriptive statistics and the evaluation of historical data.. However, with advancements in technology and an increasing availability of data, the field has evolved significantly over the years. Key milestones include the development of regression analysis in the 19th century, the introduction of data mining in the late 20th century, and the emergence of machine learning techniques in the 21st century. These advancements have allowed businesses to refine their predictive capabilities, ushering in a new era of intelligent decision-making.
Unlike traditional analytics, which typically analyzes past performance to understand what has happened, predictive analytics takes a proactive stance by forecasting future events based on existing data trends. This capability is particularly valuable in various industries, including finance, healthcare, retail, and manufacturing. For example, in healthcare, organizations can predict patient outcomes to improve treatment plans, while in retail, businesses can forecast inventory needs to optimize supply chain management. By differentiating itself from conventional analytics, predictive analytics empowers organizations to stay ahead of the curve, enabling data-driven strategies that enhance performance and growth.
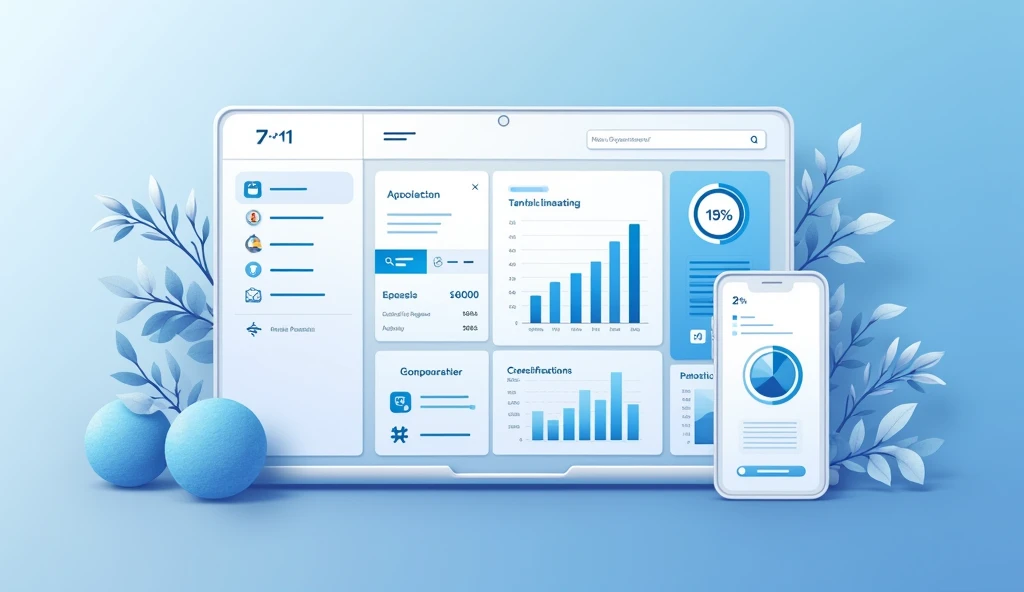
Key Techniques and Tools in Predictive Analytics
Predictive analytics is a multifaceted discipline that utilizes various techniques and tools to forecast future trends based on historical data. Among the most prominent statistical methods employed in this field are regression analysis and time series forecasting. Regression analysis, particularly linear regression, helps in understanding the relationship between dependent and independent variables, allowing businesses to predict outcomes based on influencing factors. Time series forecasting, on the other hand, is instrumental in analyzing data points collected at regular intervals, enabling organizations to project future values based on historical trends.
In recent years, the integration of machine learning techniques has transformed predictive analytics. Decision trees stand out as an approachable method for making predictions. They break down a dataset into progressively smaller subsets while developing an associated decision tree. This hierarchical structure encourages intuitive understanding and aids in decision-making processes. In contrast, neural networks serve as more complex predictive tools, mimicking the human brain’s neural connections. These algorithms excel at identifying intricate patterns within large datasets, making them particularly effective for tasks such as image recognition and natural language processing.
To facilitate the application of these techniques, a variety of software and platforms are available. Tools like R and Python offer extensive libraries, including TensorFlow and Scikit-learn, specifically designed for predictive analytics. These programming languages empower data scientists and analysts to build predictive models with advanced capabilities. Furthermore, commercially available platforms like IBM SPSS, SAS, and Microsoft Azure Machine Learning provide pre-built functions and user-friendly interfaces for businesses seeking to leverage predictive analytics without requiring extensive programming expertise.
By utilizing a combination of statistical methods and neural networks, alongside robust software solutions, organizations can harness the power of predictive analytics, driving informed decision-making and strategic planning.
Real-World Applications of Predictive Analytics
Predictive analytics has become an indispensable tool across a range of industries, enabling organizations to make data-driven decisions that drive growth and innovation. In the financial sector, banks and financial institutions use it for fraud detection, analyzing transaction patterns and identifying anomalies that may indicate fraudulent activity. This proactive approach not only minimizes financial losses but also enhances customer trust and safety.
Healthcare organizations utilize predictive analytics to improve patient outcomes and resource allocation. By analyzing patient data, hospitals can predict admission rates and identify individuals at risk of chronic diseases. This allows healthcare providers to implement preventive measures early, improving the effectiveness of treatments and optimizing the use of medical resources.
Retail businesses harness the power of predictive analytics to enhance customer segmentation and personalize marketing strategies. By evaluating purchasing behavior and demographic information, retailers can tailor their offerings to meet the needs of specific customer groups, thereby increasing engagement and boosting sales. Additionally, predictive models can forecast inventory demands, enabling businesses to maintain optimal stock levels while reducing excess inventory costs.
In the realm of marketing, organizations apply predictive analytics to better understand customer interactions and preferences. By analyzing past campaign data, businesses can predict which marketing strategies are likely to yield the highest returns and adjust their tactics accordingly. This level of insight not only improves campaign effectiveness but also enhances customer experience by delivering relevant content and offers.
Across these diverse applications, companies that have integrated predictive analytics into their operations report tangible benefits, including increased efficiency, reduced costs, and improved overall performance. The successful implementation of predictive models highlights the potential of this analytical approach to transform decision-making processes and contribute to sustainable growth in various industries.
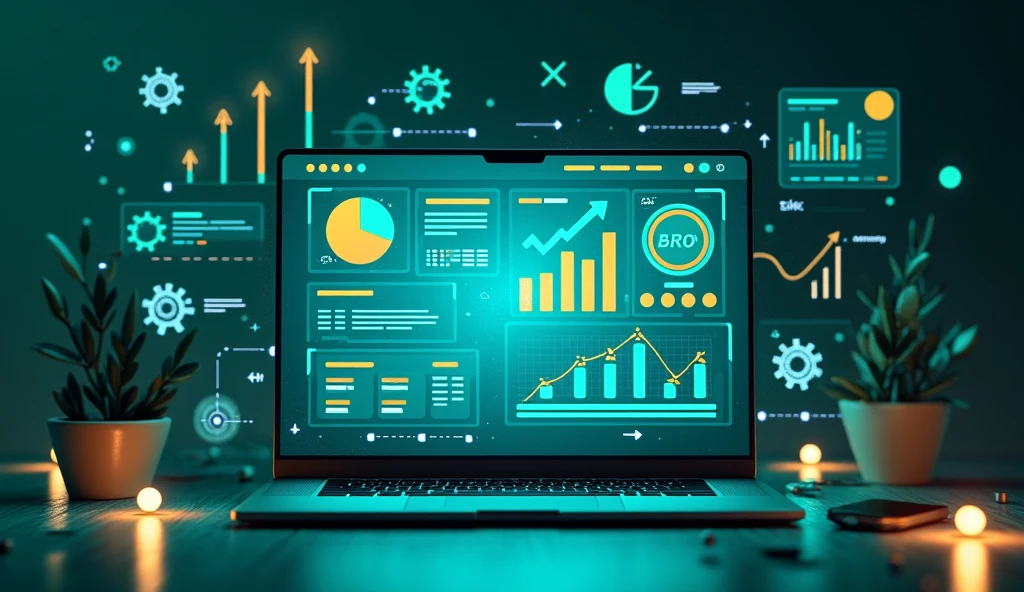
Challenges and Future Directions in Predictive Analytics
While predictive analytics offers substantial benefits for businesses seeking to enhance their decision-making processes, several challenges impede its widespread adoption. One of the primary hurdles is the issue of data quality. Predictive models rely on accurate and comprehensive datasets to generate reliable forecasts. In many cases, organizations face difficulties in cleaning and validating their data, which can lead to flawed insights and compromised decision-making. High-quality data is essential not only to improve model performance but also to build trust in the results of predictive analytics.
Another challenge lies in the need for skilled personnel. The successful implementation of predictive analytics demands not only technical expertise in data science and statistics but also a deep understanding of the specific business context. There exists a significant skill gap in the workforce, as many organizations struggle to find experts who can navigate complex data environments and interpret results effectively. Consequently, investing in training programs and fostering a culture of continuous learning is vital for companies aiming to leverage predictive analytics.
Ethical considerations also warrant attention in the realm of predictive analytics. As organizations increasingly rely on algorithms to inform decisions, issues surrounding bias, privacy, and transparency come to the forefront. Stakeholders must develop frameworks to ensure ethical data practices and mitigate risks associated with algorithmic decision-making. This involves embedding ethical review processes within the analytics workflow to ensure fairness and accountability.
Looking ahead, the future of predictive analytics is promising, with anticipated developments including the integration of artificial intelligence and big data technologies. As organizations continue to harness the capabilities of machine learning and AI, predictive models will become more sophisticated, enabling deeper insights and greater accuracy. Additionally, the growth of big data will provide access to a wealth of information that can further enhance predictive capabilities, allowing businesses to stay ahead in an increasingly competitive landscape.
Conclusion
Predictive analytics has emerged as a transformative tool, empowering organizations across industries to make informed decisions and anticipate future outcomes with precision. By leveraging statistical techniques, machine learning algorithms, and advanced tools, businesses can derive actionable insights that drive growth, enhance efficiency, and improve customer experiences.
Despite its immense potential, predictive analytics is not without its challenges. Issues related to data quality, skills gaps, and ethical concerns must be addressed to ensure reliable and responsible implementation. However, as technology advances and organizations adopt more sophisticated methods, its future looks bright. Its integration with artificial intelligence and big data promises even greater accuracy and innovation, enabling companies to navigate complexities and remain competitive in a rapidly evolving world.
Ultimately, predictive analytics represents a vital component of modern strategic planning, offering the foresight needed to thrive in today’s data-driven economy.
FAQ: Predictive Analytics
What is it?
It involves using statistical techniques, machine learning, and historical data to predict future outcomes, identify trends, and support decision-making across a variety of industries.
How does it differ from traditional analysis?
While traditional analytics focuses on analyzing past performance, predictive analytics forecasts future outcomes based on patterns and trends found in historical data.
What are the main benefits?
Helps organizations improve decision making, optimize operations, reduce costs, enhance customer experiences, and gain competitive advantage.
Which industries benefit the most from predictive analytics?
It is widely used in finance (fraud detection), healthcare (predicting patient outcomes), retail (inventory forecasting), and marketing (personalized campaigns).
What are the main techniques used?
Common techniques include regression analysis, time series forecasting, decision trees, and neural networks, often supported by machine learning tools.
What tools are used in predictive analytics?
Popular tools include programming languages like Python and R, with libraries such as TensorFlow and Scikit-learn, as well as platforms like IBM SPSS, SAS, and Microsoft Azure Machine Learning.
What are the main challenges?
Key challenges include ensuring data quality, addressing skill gaps in data science, and navigating ethical concerns like bias, privacy, and transparency.
How does predictive analytics handle data quality issues?
Organizations must invest in robust data cleaning and validation processes to ensure datasets are accurate, consistent, and comprehensive for reliable model predictions.
What role does ethics play in predictive analytics?
Ethics in predictive analytics involves addressing bias in algorithms, maintaining data privacy, and ensuring transparency in decision-making processes.
What is the future of predictive analytics?
The future includes deeper integration with artificial intelligence and big data, enabling more sophisticated models, greater accuracy, and innovative applications across industries.